Predictive analytics in the context of safety management
Key Points in this blog post
- Predictive analytics uses historical data to anticipate future safety incidents, leading to proactive safety management.
- Procore, a construction management software, aids in utilizing predictive analytics for safety.
- Implementations of predictive analytics, like at Goldcorp, have led to substantial reductions in injury rates.
- Despite its benefits, predictive analytics implementation faces challenges such as data privacy, security, and quality.
- Future advancements in AI, IoT, and wearable sensors will enhance the precision of predictive analytics in safety.
- The benefits of predictive analytics include improved safety, cost reduction, and increased employee morale.
- Organizations are encouraged to embrace predictive analytics to ensure workforce safety and stay competitive.
The evolution of AI technology and data collection capabilities has birthed an era where predicting future outcomes based on historical and real-time data isn't just feasible; it's rapidly becoming the norm. This is especially crucial in the context of safety management, where the right prediction could literally mean the difference between life and death.
Enter predictive analytics, a branch of advanced analytics, utilizing various techniques such as data mining, statistics, modeling, machine learning, and artificial intelligence to analyze current data and historical facts to forecast future events.
Predictive analytics holds significant relevance for safety management, an area traditionally dominated by after-the-fact analysis and reactionary measures. It offers the ability to foresee potential safety hazards and incidents before they occur, thus enabling preventive measures to mitigate risks effectively. Predictive analytics in safety management isn't about merely responding to accidents but, more importantly, preventing them from occurring in the first place.
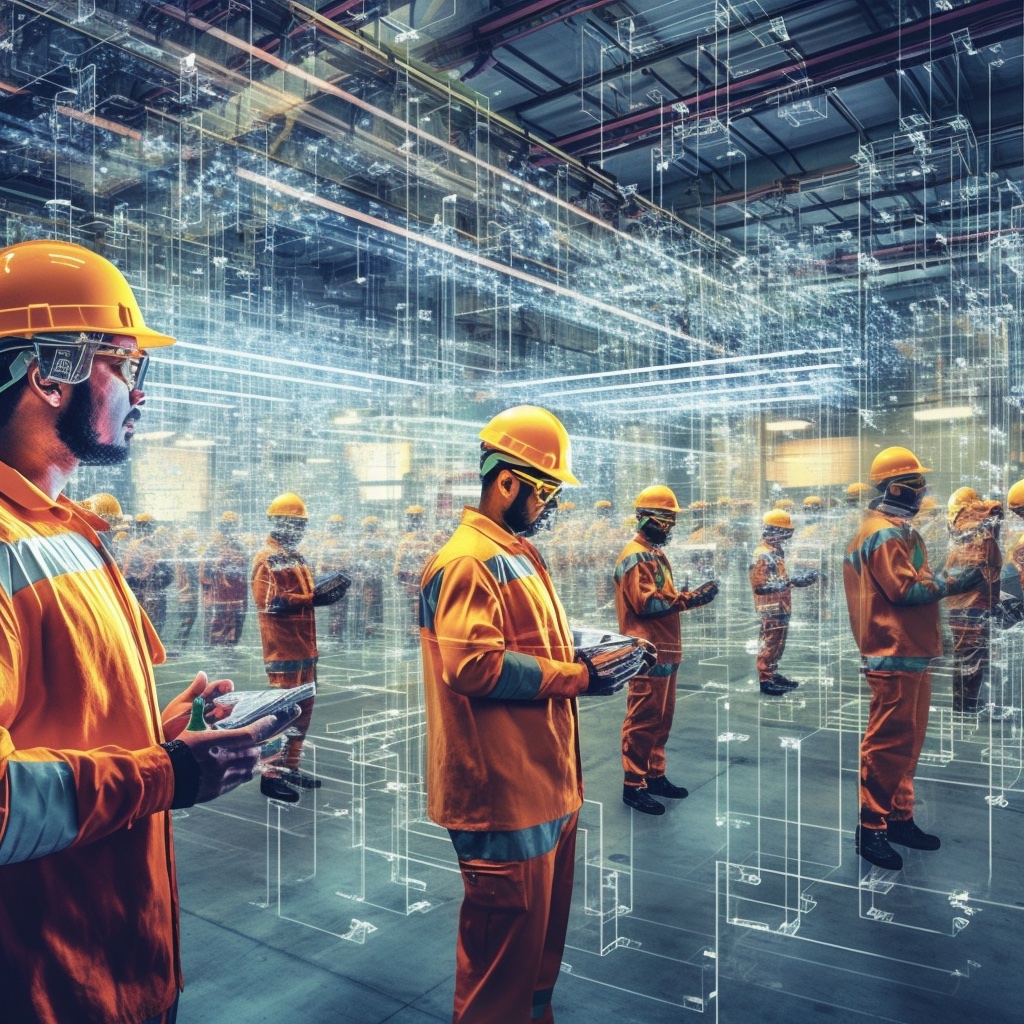
Predictive analytics can save lives
By analyzing patterns in data collected from various sources, such as sensors, cameras, and workers' wearable devices, predictive analytics can help identify potential risks or dangerous behaviors. From detecting machinery that's likely to fail, spotting hazardous behaviors to predicting high-risk scenarios based on environmental conditions, predictive analytics can save lives by enabling timely interventions.
The power of predictive analytics, however, doesn't stop at mere predictions. It also enables organizations to prioritize safety initiatives based on risk levels, optimize resource allocation, and drive a proactive safety culture. This paradigm shift in safety management holds the potential to significantly reduce workplace accidents and fatalities, while also contributing to improved operational efficiency and cost savings.
In the subsequent sections, we'll delve deeper into the role of predictive analytics in safety management, explore some real-world case studies, examine the challenges of implementation, and glimpse into the future of this transformative approach to workplace safety.
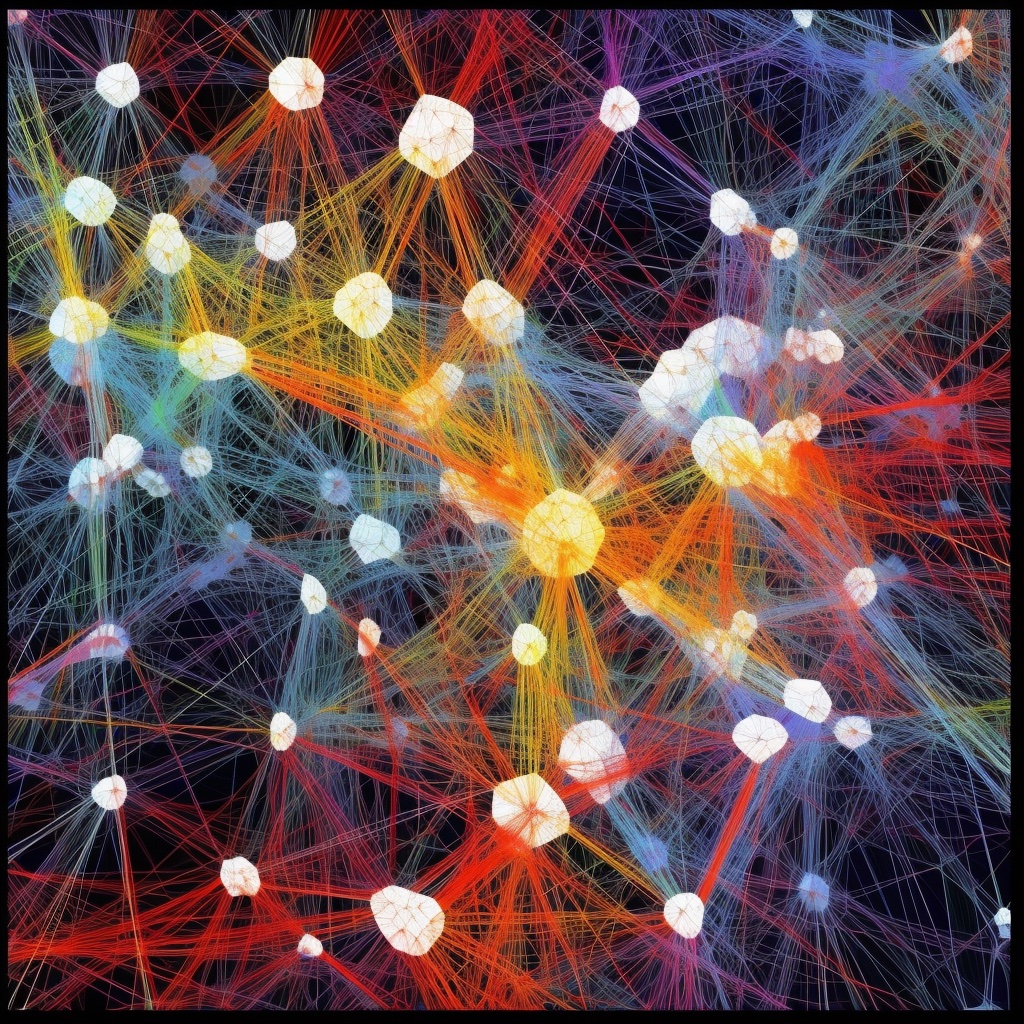
Understanding Predictive Analytics
- A simple, layman's explanation of what predictive analytics is
- Various types of data used in predictive analytics for safety
Predictive analytics is a powerful tool in today's data-driven society. Defining it is deceptively simple. According to IBM, it is a branch of advanced analytics that simply allows us to make predictions about future outcomes. How does it do this? It uses historical data combined with statistical modeling, data mining techniques, and machine learning. The aim of predictive analytics is to find patterns within the data that can help us identify risks and opportunities.
In the realm of safety management, predictive analytics plays a pivotal role. To understand how, let's first delve deeper into the kind of data we feed into this analytical machine.
Which types of data are used in predictive analytics for safety
There is a wealth of information captured in historical worksite data, project reports, human resource records, inspection reports, and environmental data. When this data is run through predictive models, patterns begin to emerge, painting a clearer picture of potential hazards before they manifest into real problems. Think of it as a sophisticated warning system; it's about harnessing the power of data to foresee potential incidents and strategically intervene before they occur.
For instance, risk predictors, such as project site, project size, team size, weather condition, and project phase can all be used to predict safety issues. These predictors are independent and quantitative. When analyzed accurately, they provide us with insights into high-risk areas or employee groups that might need additional safety resources or wellness programs.
Imagine a construction site where the team size is large, the project phase is at its peak, and the weather condition is challenging. The site team may be aware of this, but aggregated across the entire business, where is the riskiest activity occurring at any one point in time? Predictive analytics can flag such scenarios as high-risk, prompting necessary precautionary measures to ensure the workers' safety.
Beyond risk predictors, predictive analytics also uses incident and accident data, project status, near-miss data, workplace data, and employee data. All these data points, when combined, present a holistic view of the organization's safety status. It's like piecing together a puzzle; each data point provides a small piece of the larger safety picture. By understanding the story that the data tells, we can make proactive, informed decisions that prioritize safety.
At its core, predictive analytics empowers companies to not just react to safety incidents after they occur, but to proactively prevent them. It's a shift from a reactive safety approach to a preventive one, transforming the way organizations handle safety management.
Embracing predictive analytics means stepping into a future where safety-related objectives are not only achieved but exceeded. Safety Risk Predictive Analytics can enhance employee satisfaction, reinforce employer corporate responsibility, and ultimately, lead to a safer, more sustainable organization.

The Role of Predictive Analytics in Safety Management
- A deep dive into the role of predictive analytics in preventing accidents and enhancing safety measures
- Discussion on the advantages of data-driven safety measures over traditional methods
- Real-life examples where predictive analytics has been used to improve safety and save lives
As technology continues to evolve, the landscape of safety management is experiencing a seismic shift. Traditional methods of analyzing incidents after they occur are now being replaced with more proactive approaches. And at the forefront of this shift is predictive analytics.
Predictive analytics brings a fresh perspective to safety management. The role of predictive analytics here is multifaceted; it aids in early hazard identification, improves safety performance, increases productivity, and reduces costs associated with accidents.
The early identification of hazards is a game-changer. By analyzing data trends and patterns, predictive analytics identifies potential hazards before they materialize into accidents. This foresight enables safety managers to initiate preemptive measures - whether it's additional training for the workforce, installing new safety equipment, or revising existing procedures.
The ripple effect of this predictive power is tangible. Improved safety performance is an inevitable outcome, marked by a reduction in accidents, injuries, and illnesses. This can translate into lower insurance premiums, a direct financial benefit for the company. Moreover, a safer work environment leads to increased productivity. When employees feel safe and secure, they can focus more on their roles, leading to improved performance and productivity.
The financial implications of predictive analytics in safety management can't be overstated. Accidents incur hefty costs in terms of medical expenses, legal fees, and lost productivity. By mitigating the number of accidents, predictive analytics can significantly save businesses money.
There are real-world instances where predictive analytics has saved lives. A shining example is the global construction company, AECOM. In 2020, they leveraged predictive analytics to reduce worker injuries by 15%. Their predictive analytics platform identified workers who were at a high risk of injury - particularly those new to the job. As a result, they provided these individuals with additional training and support, preventing potential accidents.
Moreover, safety analytics are being implemented in the healthcare sector. Hospitals use predictive analytics to anticipate patient deterioration, enabling timely interventions. This prevents complications, reduces mortality rates, and improves patient outcomes.
Thus, predictive analytics serves as a lighthouse for safety management. It shines a light on potential hazards, guiding safety managers to take informed, proactive measures. The power to foresee risks and prevent them is changing the safety landscape, creating safer environments, enhancing productivity, and saving lives. It's not just about managing safety anymore; it's about predicting and preventing it.

Procore and Predictive Analytics
- Introduction to Procore, its features, and its role in safety management
- A step-by-step guide on how to use Procore for predictive analytics
Procore Login: Your Gateway to Predictive Analytics
In the quest for a safer and more productive workplace, technology platforms such as Procore have emerged as crucial tools in leveraging the power of predictive analytics. Procore, a cloud-based construction management software, is designed to streamline processes, improve communication, and provide data-driven insights that lead to safer, smarter decision-making.
Much like how a simple credit card transaction triggers a series of predictive analyses—like assessing whether the purchase is typical for you or if the card is being used in a common location—Procore operates behind-the-scenes to enhance safety management. It does this by analyzing various types of data to forecast potential safety issues, thereby allowing companies to take preemptive measures.
So, how exactly can you use Procore for predictive analytics in safety management? It starts with the understanding that traditional methods, such as analyzing past accident reports, only provide “lagging indicators.” Predictive analytics, on the other hand, requires focusing on the factors that cause incidents.
After logging into Procore, you can input and access various types of data, including vehicle and human telemetry, geospatial data, and equipment telematics. You can also incorporate data from everyday business transactions, such as payroll and performance information, social and demographic data, and training records. These can all be valuable predictive factors in forecasting potential safety incidents.
Procore's predictive analytics capabilities can assist you in identifying which tasks under specific conditions increase the likelihood of injury. Suppose a particular tool used by carpenters frequently results in shoulder injuries when an alternative isn't available. With these insights, you could find alternative tools or processes, significantly improving the well-being of your teams.
This increased safety consciousness isn't just theoretical; researchers at Carnegie Mellon University built predictive models using real workplace safety data that could predict the number of injuries a job site would have with impressive accuracy rates of 80 to 97%.
Additionally, smart devices can be incorporated with Procore to further enhance safety measures. An example is Caterpillar's Cat Smartband, which alerts wearers and managers when operators lack sleep and are prone to fatigue. This sort of integration is where Procore shines - its ability to amalgamate various data sources into a single, accessible platform.
The bottom line is that Procore, when used to its full potential, can propel your safety management into a predictive realm. It can provide a safety dashboard with insights that not only anticipate safety issues but also highlight where your preventive efforts are yielding results. This transition to predictive analytics, achievable through your Procore login, can lead to lower insurance premiums, reduced claims costs, and most importantly, a safer workplace.

Case Study: Predictive Analytics in Action
- Detailed case study of a company/industry that has successfully used predictive analytics for safety management
- Analysis of the case study: what worked, what didn't, and why
Our journey into the practical implementation of predictive analytics for safety management takes us to the operations of two industry giants: the multinational construction firm, AECOM, and the mining company, Goldcorp Inc.
At AECOM, predictive analytics was leveraged to reduce worker injuries by 15%. The firm utilized a predictive analytics platform to identify workers who were at an elevated risk of injury. The model predicted that new workers were more likely to get injured, prompting the company to provide additional training and support to these workers. AECOM's experience showcases the potential of predictive analytics in identifying high-risk groups and taking preventative action to ensure their safety.
Goldcorp Inc. provides another success story in the use of predictive analytics. Over a six-year period, Goldcorp managed to decrease injury frequency rates by a remarkable 75% by implementing analytics services. The company drew on the wealth of data available to them, including accident and incident history, equipment usage, work conditions, and more, to feed their predictive analytics model.
The successes of AECOM and Goldcorp can be attributed to several key factors. Firstly, both companies collected and used high-quality data. Without accurate, relevant data, predictive analytics is meaningless. Both companies had a wealth of data at their disposal, which provided a solid foundation for their predictive models.
Secondly, both firms recognized the importance of focusing on high-risk predictors and lead indicators of safety issues. By identifying these factors, they were able to take targeted action to mitigate risks, whether that meant providing additional training, adjusting work procedures, or addressing potential hazards.
Finally, these companies demonstrated a willingness to evolve and adapt their safety strategies based on the insights provided by predictive analytics. They didn't just use predictive analytics to identify risks; they also took action based on those insights. This proactive approach to safety management is perhaps the most critical element of their success.
However, it's important to note that implementing predictive analytics is not without challenges. One potential difficulty lies in ensuring data quality and availability, as the efficacy of predictive analytics is heavily dependent on these factors. Additionally, predictive analytics models can be complex, making it difficult for organizations to understand and effectively utilize the results.
Nonetheless, as shown by the cases of AECOM and Goldcorp, the benefits of predictive analytics in safety management are clear: fewer accidents, increased productivity, and significant cost savings. These successes suggest that predictive analytics has the potential to revolutionize safety management across industries, paving the way for a safer, more productive future.

Challenges in Implementing Predictive Analytics for Safety Management
- The common barriers and challenges that organizations face while adopting data-driven safety practices
- Tips on how to overcome these challenges
The implementation of predictive analytics in safety management, though rewarding, is not devoid of challenges. From data quality concerns to model complexity and stakeholder acceptance, organizations embarking on this data-driven journey may face numerous hurdles. Let's dive into these challenges and discuss strategies to overcome them.
Perhaps the most fundamental challenge lies in the realm of data. Good quality data is the backbone of any successful predictive analytics initiative. However, ensuring data quality and availability can prove to be a daunting task. Data can be incomplete, outdated, inconsistent, or even incorrect, undermining the reliability of the predictive models. Moreover, companies often struggle with data silos, where valuable information is confined within specific departments, inhibiting a holistic view of safety.
To tackle these issues, organizations should adopt a robust data management strategy, promoting data integrity, consistency, and accessibility. A culture of data sharing and transparency can also help break down silos, allowing for a comprehensive approach to safety management.
Model complexity is another common roadblock. Predictive analytics models, especially those based on advanced machine learning techniques, can be intricate and hard to understand. This complexity can pose difficulties in interpreting the model's outcomes and integrating them into safety strategies.
To overcome this, organizations can seek help from data scientists or use interpretable machine learning models. Furthermore, investing in training to improve the data literacy of safety managers can also be beneficial, enabling them to better understand and utilize the results of predictive analytics.
Another significant challenge is stakeholder acceptance. Like any new technology, predictive analytics can meet with resistance. Stakeholders may express concerns about the accuracy of the predictive models, the costs of implementation, or the potential impact on their roles. To mitigate this, it's important to manage change effectively. This involves clear communication about the benefits of predictive analytics, training programs to ease the transition, and a gradual, phased approach to implementation.
Finally, privacy and ethical concerns can arise when collecting and using employee data for predictive analytics. It's crucial to respect individual privacy rights and follow applicable data protection laws. Providing transparency about the use of data and implementing strong data security measures can help build trust and ensure ethical use of data.
In conclusion, while the journey to implementing predictive analytics in safety management is filled with challenges, they are not insurmountable. With a strategic approach focused on robust data management, continuous learning, effective change management, and a strong ethical foundation, organizations can successfully navigate these challenges and harness the power of predictive analytics to enhance their safety performance.
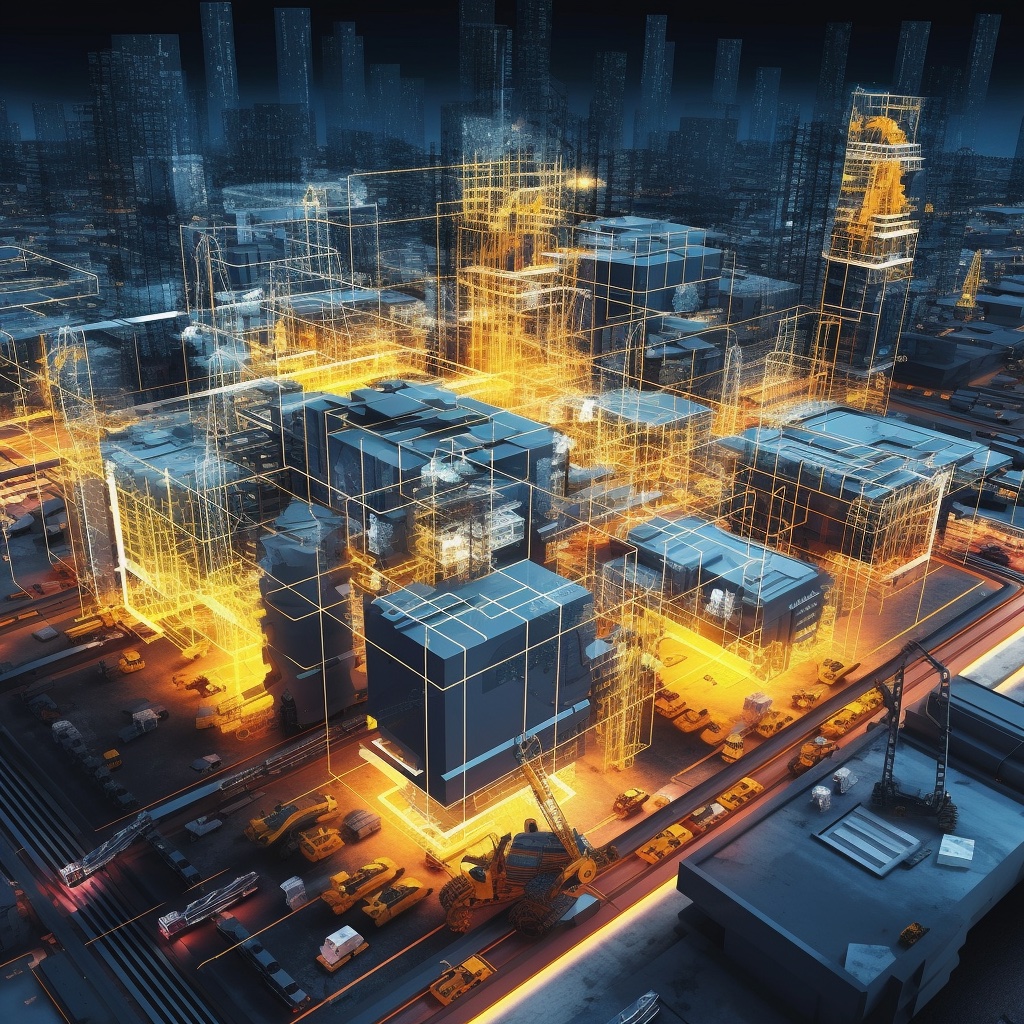
The Future of Data-Driven Safety
- Predictions about the future of predictive analytics in safety management
- How companies can prepare for these changes
In the continuously evolving landscape of occupational safety, data-driven methodologies and predictive analytics stand out as revolutionary elements. Current trends highlight an increasing adoption of wearable sensors, Internet of Things (IoT) devices, and machine learning algorithms in safety management, which offer significant potential in proactively reducing workplace accidents and injuries. These technological innovations, when coupled with the rewarding of risk-reducing behaviors, are empowering organizations to adopt a more proactive and efficient approach to safety management.
The use of augmented and virtual reality in safety training has also been a game changer. It not only provides a safe and controlled environment for workers to learn and make mistakes, but also significantly improves the ability to identify potential hazards. Another key trend is Workforce Analytics, which leverages data related to employee behaviors, training, and performance to foresee potential safety risks and craft proactive safety interventions.
However, adopting predictive analytics in safety management is not without its challenges. Key among these is maintaining data quality and security, a concern being increasingly recognized by regulators who are focusing on developing new regulations and requirements that address these unique challenges.
Interestingly, the field of safety management is experiencing a paradigm shift with the use of AI, Machine Learning, and IoT technologies. More sophisticated machine learning algorithms are improving the ability to identify patterns and trends in data, and this trend is likely to continue. It's expected that the adoption of predictive analytics for safety management, which was at 15% in 2017, would rise to 35% by 2022 according to a study by the Aberdeen Group.
Moreover, with the advent of chat-based AI and large language models like ChatGPT, user interaction with predictive analytics is likely to become more seamless and intuitive. This means that it's not just the answers or results from the analytics that matter, but also the questions posed to the AI. The ability to prompt AI effectively to yield insightful predictive analytics will be a key skill in the future.
In terms of regulatory dynamics, requirements for data quality validation and data retention, as well as data security, such as data encryption and data access controls, are likely to become more stringent. Moreover, ethical implications, including potential bias and discrimination, are expected to come under regulatory scrutiny.
Big data is also playing a vital role in reshaping safety management. Its ability to identify high-risk areas, predict potential hazards, monitor worker behavior, and enhance communication allows for the creation of a safer and more productive work environment.
The field of safety management, consequently, is poised for a transformation, driven by predictive analytics. By embracing these changes and investing in these technologies, companies will not only make their workplaces safer but also foster a culture that values safety, ultimately contributing to a positive impact on employee morale, productivity, and overall business performance.

Conclusion
- A summary of the key points discussed in the blog
- A strong call-to-action encouraging readers to embrace predictive analytics in their safety management strategies
Predictive analytics is poised to play an instrumental role in revolutionizing safety management strategies. Through the collection and analysis of vast amounts of data, predictive analytics can accurately forecast potential safety hazards, enabling companies to address issues before they evolve into critical incidents.
Embracing such data-driven strategies allows organizations to replace traditional reactionary approaches with proactive, preventive measures, considerably enhancing safety standards. Case studies like Goldcorp Inc.'s successful deployment of predictive analytics, leading to a 75% drop in injury frequency rates, underscore the significant impact and potential of this technology in fostering safer work environments.
However, the path to integrating predictive analytics into safety management is not devoid of challenges. Concerns regarding data quality, security, and ethical implications, as well as a steep learning curve for machine learning and AI technologies, are significant hurdles that organizations may encounter. However, with careful planning, leveraging outside help when necessary, and a commitment to continuous learning, these obstacles can be successfully navigated.
The future of safety management is data-driven. Rapid advancements in technologies such as AI, Machine Learning, IoT, and sophisticated wearable sensors, coupled with the increasing adoption of AR and VR for safety training, signal a shift towards an era where safety measures are not just enforced but intelligently anticipated.
Therefore, the call-to-action is clear. It's time for organizations to embrace predictive analytics in their safety management strategies. This isn't merely about keeping up with the times or embracing the latest tech trends, but about creating safer workplaces, protecting valuable human resources, improving productivity, and ultimately, driving business growth.
So, step into the future of safety management. Invest in predictive analytics, champion a culture of safety, and transform your workplace into a safer, more productive, and more rewarding space for everyone.
FAQ
Q: What is predictive analytics in the context of safety management?
A: Predictive analytics in safety management involves using data, statistical algorithms, and machine learning techniques to identify the likelihood of future outcomes based on historical data with the aim of preventing accidents and improving safety measures.
Q: How does predictive analytics improve safety measures?
A: Predictive analytics can help identify patterns and trends in data, which can in turn help organizations anticipate and prevent potential accidents and hazards. This results in a more proactive approach to safety and can contribute to a significant reduction in workplace accidents.
Q: How can Procore help in safety management using predictive analytics?
A: Procore's robust safety management platform allows organizations to leverage predictive analytics by aggregating safety data from various sources, analyzing trends and patterns, and providing actionable insights to prevent accidents and enhance workplace safety.
Q: Can you provide examples of industries that have successfully used predictive analytics in safety management?
A: Goldcorp, a mining company, used predictive analytics services and reported a 75% drop in injury frequency rates over six years. Additionally, companies like Deloitte and Caterpillar have developed products to analyze multiple factors leading to workplace safety incidents.
Q: What are the challenges organizations may face while implementing predictive analytics in safety management?
A: Organizations may face challenges in terms of data privacy and security, data quality, integration of data from different sources, a lack of skilled professionals, and the need for a cultural shift to embrace data-driven safety practices.
Q: How can organizations overcome these challenges?
A: Organizations can overcome these challenges by implementing stringent data privacy and security measures, ensuring data quality, investing in the right technology and professionals, and fostering a data-driven culture through proper training and education.
Q: How is the future of predictive analytics shaping in safety management?
A: The future of predictive analytics in safety management is promising with developments in AI, machine learning, IoT, wearable sensors, augmented reality, and virtual reality. This technology will enable even more precise prediction and prevention of safety risks.
Q: What are the benefits of using predictive analytics in safety management?
A: Benefits include improved safety through early identification and prevention of hazards, reduced costs associated with accidents and injuries, increased employee morale, better decision-making, and a more proactive approach to safety management.